Peptides are short proteins. For proteins, one typically envisions a long chain of amino acids that has folded up on itself to form a compact blob that is capable of performing some function, like catalyzing a reaction. Peptides are often less folded and their main function is to bind other proteins. You can see examples of both below.
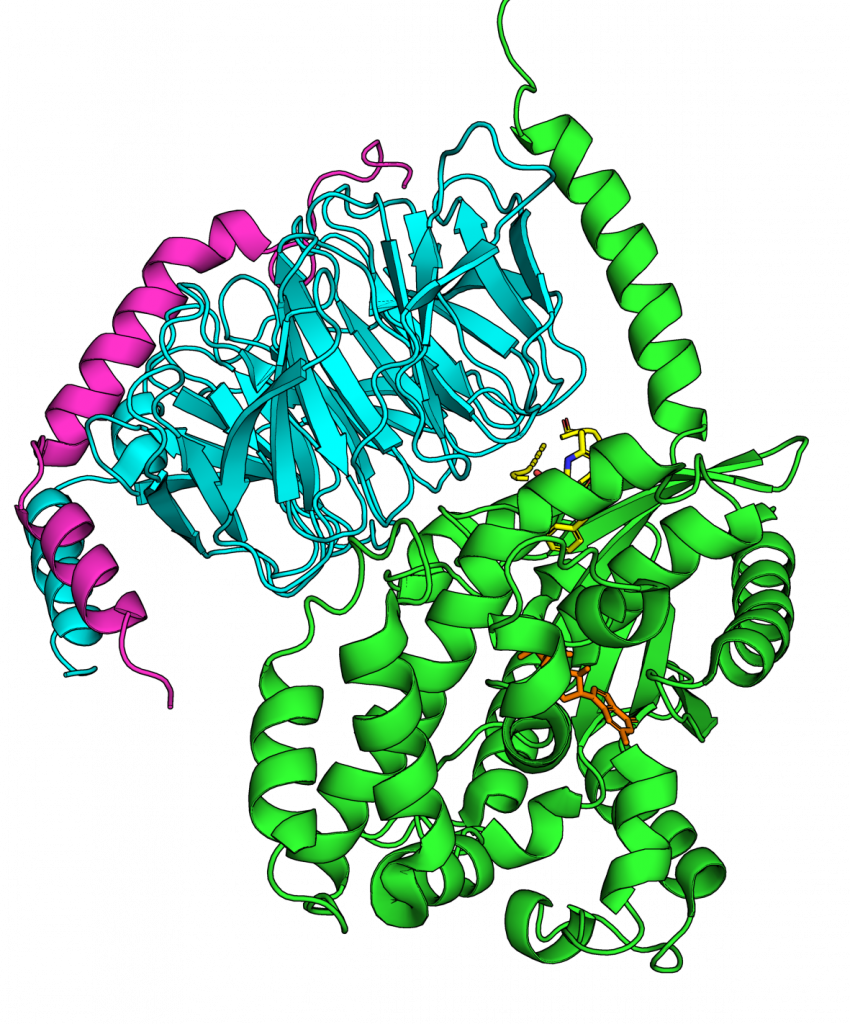
Historically, the pharmaceutical industry has avoided peptides. Our bodies have lots of mechanisms for breaking up peptides and it wasn’t clear that peptides could get into cells. In contrast, small molecule drugs are quite stable in the human body and can pass through membranes.
However, there is now growing interest in peptide-based drugs. Scientists have come up with a variety of ways to keep them from getting chewed up. They have also devised a number of ways to get peptides into cells.
Moreover, peptides are great at binding parts of proteins that are difficult to target with small molecules drugs. Small molecules are well-suited to binding in pockets on a protein’s surface. However, it’s hard to get them to bind tightly enough to flat interfaces. Peptides are much better at this (see figure above for examples).
Machine learning (ML) is also having a profound impact on peptide and protein design. Peptides and proteins are made of linear chains of amino acids, with each link in the chain being one of twenty different possibilities. The scientific community has a lot of data on protein sequences, the structures they form, and the other things they bind to. Pairing this wealth of data with advances in ML is allowing unprecedented successes in protein and peptide design. In contrast, there are an essentially infinite number of different ways to arrange atoms into a small molecule drug. While scientists have lots of data on small molecules, the amount of data per possible compound is far more limited and ML methods struggle more than for peptides/proteins.
For more, you can read this recent review from the Shukla group.