Now that Folding@home has turned 21, we are revisiting some classic papers from the team that have had significant scientific impacts. Sorin and Pande (2005) describes simulations of alpha-helix folding: i.e. the helix-coil transition.
Exploring the helix-coil transition via all-atom equilibrium ensemble simulations
EJ Sorin, VS Pande
Biophysical journal 88 (4), 2472-2493
Why is this work important?
When Folding@home (FAH) debuted in 2000, the new insight it promised was an ability to observe rare folding events through parallel, crowdsourced simulations. This paper focused more on the unprecedented statistical precision that can be achieved with FAH. Unlike previous studies analyzing a handful of trajectories of alpha helix folding, Sorin and Pande used FAH to perform thousands of simulations with lengths on the order of ~100 ns, starting from both unfolded and folded states. This produced an ensemble of trajectories that completely converged to thermodynamic equilibrium, enabling quantitative comparison to equilibrium experimental measurements of the helix-coil transition.
I recently got a chance to talk with Eric Sorin, who is now a Professor of Chemistry at California State Long Beach, to talk about his now-classic paper.
Vincent: Hi Eric, thanks for taking the time to talk with me. Do you have general thoughts on the impact and importance of this paper? Other than the 2002 Nature paper published after Folding@home’s debut, I believe it’s the most-cited scientific paper from Folding@home, with over 740 citations.
Eric Sorin: Yeah, it’s definitely the crown jewel in the collection of papers [I published] as a grad student. I did an extended stay at Stanford, so it was like grad school and a postdoc back to back. I think its impact is based on the notion that we could really start doing equilibrium simulations. It got a lot of people thinking about the question of how—either through massively parallel computing, or high performance computing with longer simulation times—can we reach conformational equilibrium? I’m almost certain—both at the time, and now—that this was the first paper where that was actually achieved and published.
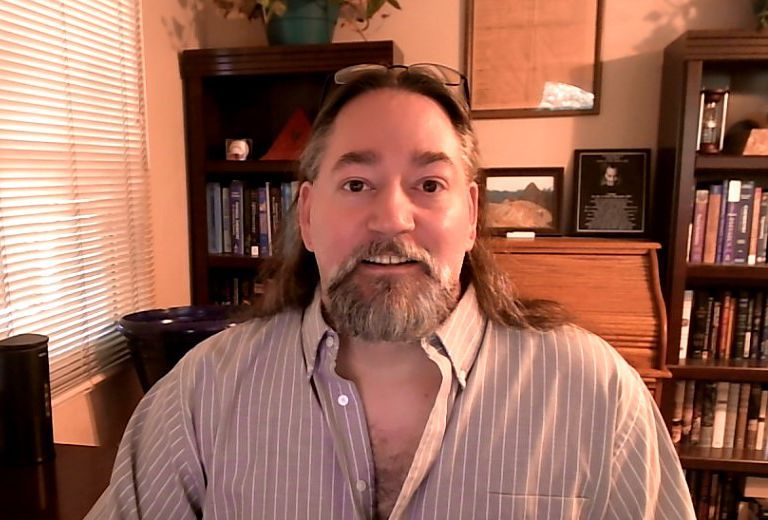
It is remarkable all things you were then able to do with all that equilibrium sampling. For one, you were able to make very precise comparisons with experiments to evaluate different force fields.
Yeah, to really truly analyze the accuracy of different force fields [from equilibrium sampling], I think that was the first time that was done.
Could you say a bit about the Lifson-Roig model (1), and how you used it to compare your simulations to experiment?
Well, you know, I have to partially thank David Chandler. This was when he was still at Berkeley. He was not supposed to let us know, but he turned out to be one of the referees. And he put me through about three months of hell, pushing to make that paper better and better and better!
That was the only time I ever worked with him; I’ve never had an experience like that. He was communicating with me through Vijay [Pande] and basically telling us what he’d really like to see in the manuscript. David definitely pushed me to improve the original paper, where I think a lot of other people would have just let it slide. He helped me turn it into a much, much higher-caliber paper, due to his feedback.
I have to say, I reference this paper all the time. I think it’s one of the clearest explanations of Lifson-Roig helix-coil theory I’ve seen. When I re-read it, I also was reminded that a lot of the experimental measurements came from the great Robert “Buzz” Baldwin, who was active at Stanford then. Did you ever talk with him about your work?
Yeah, I met with Buzz. It wasn’t good, to be honest [laughs]. At the time, I was already kind of a long-haired hippie freak, and Buzz was a very clean-cut conservative guy, and immediately, we did not really mesh.
One of the things that I was doing was trying to compare my results to generic CD [circular dichroism] data and he had a really big problem with it, because maybe he thought I was discounting CD data, or understating the importance of CD data and the difference between the CD data and what I was seeing. Interestingly enough, we only had the one meeting.
Later, when I was on the interview circuit, I had lots of discussions with people who felt strongly that CD was being misinterpreted, especially for short helical stretches that could be highly transient. I don’t think these questions ever got resolved, and I still have questions about what happens when you’re working with a very small segment. But yeah, Buzz was a ball-buster. He definitely gave me a hard time, but it was interesting nonetheless because he was one of the great experimental masters studying helix formation.
Another aspect of the paper, one that I had kind of forgotten, was your work comparing simulated and experiment folding kinetics. You even built one of the earliest Markov models, a harbinger of all of the MSMs of protein folding that were soon to come from the Pande Lab.
I had audited a stochastics class through the statistics department, and learned about Markov chains and Markov models, just sitting in and listening. It really was like, “oh, this is very much what we’re doing”. At the time, I don’t even think I used the word “Markov model” in the paper. But yeah, that was basically the inspiration; trying to identify all of these different local energy minima and how they connected. It was very much an early Markov model, just without that label.
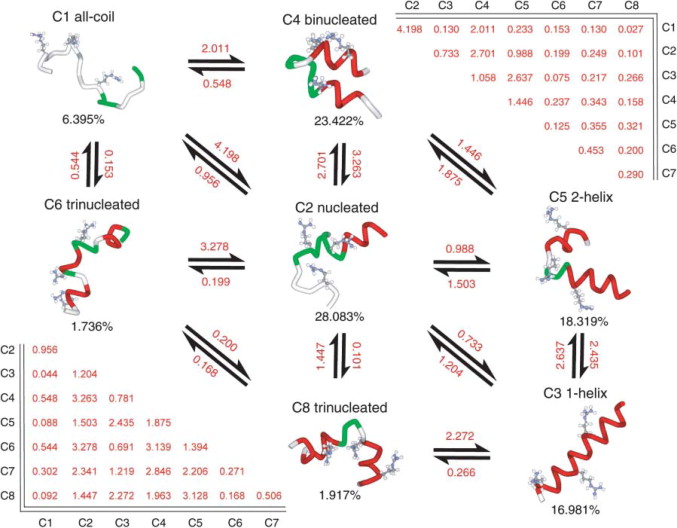
Yet another remarkable aspect of the paper was that you were able to perform a thorough comparison of many different AMBER force fields. You even proposed a new force field variant, and tested it.
That’s a little sticky [laughs]. That is certainly how we presented it. But to be brutally honest, it was sheerly accidental. During the porting of the AMBER force field to GROMACS, I accidentally mixed the backbone torsions from one force field with another [the AMBER 94 torsions with the AMBER 99 force field]. And, it turned out—at least for the alanine-rich helices we simulated—it did the best job! It turned out to perform poorly for proteins with beta-sheet structure, but it’s one of the force fields I still use and test in my current work.
I think it was also the first time that a non-AMBER research group published an AMBER variant. This has since become the standard—there are now like eight different variants, or something.
Eric Sorin
The idea of using distributed computing for force field validation remains a hot topic today. I can think of several efforts underway to do this on an ongoing basis for small-molecule force fields, for example. To what extent do you feel your work in 2005 set the stage for this work?
I’ve seen a lot of AMBER variant papers published, but I don’t think anybody’s come close to the sampling. Perhaps people are coming close, though; I haven’t been looking at those papers in the last few years. Force field validation and testing is certainly much easier to do today using high-performance computing resources than it was 15 years ago. It’s interesting to see the directions in which people have taken it. I feel like it’s really moved forward in the last 15 or 16 years since then, and probably will continue to do so.
It’s really interesting to contrast the early Folding@home work, where the focus was more on basic science questions, with today’s work, where the mission is more intentionally aimed at treating and understanding disease, and computational drug discovery.
In those early days, we talked a lot about the mission [to cure diseases], but I don’t think any of that happened right away. I mean, I was Vijay [Pande]’s, like, third student. So, he was just starting his second year [as an Assistant Professor] and at that point, Folding@home was going to come out the following year. In those early days, the mission [of curing diseases] was much more easy to spin. It was the direction we were going to go, but I don’t think it really happened, not at least while I was there.
It’s really cool to see that Folding@home is moving more toward that early mission, contributing to medical science in this way, and see it actually happening.
(1) The Lifson-Roig model is a statistical-mechanical model describing the probability of the amino acid residues in an alpha helix to be in a particular pattern of helix vs. coil conformations. There are two main parameters of this model: one to describe the propensity of helix nucleation, and the other describing the propensity of elongation for a helix that has already been templated. Like larger proteins, alpha-helices often fold cooperatively (in an all-or-nothing fashion); this is because it is unfavorable to nucleate a helix, but once it has been nucleated, elongation is favorable.