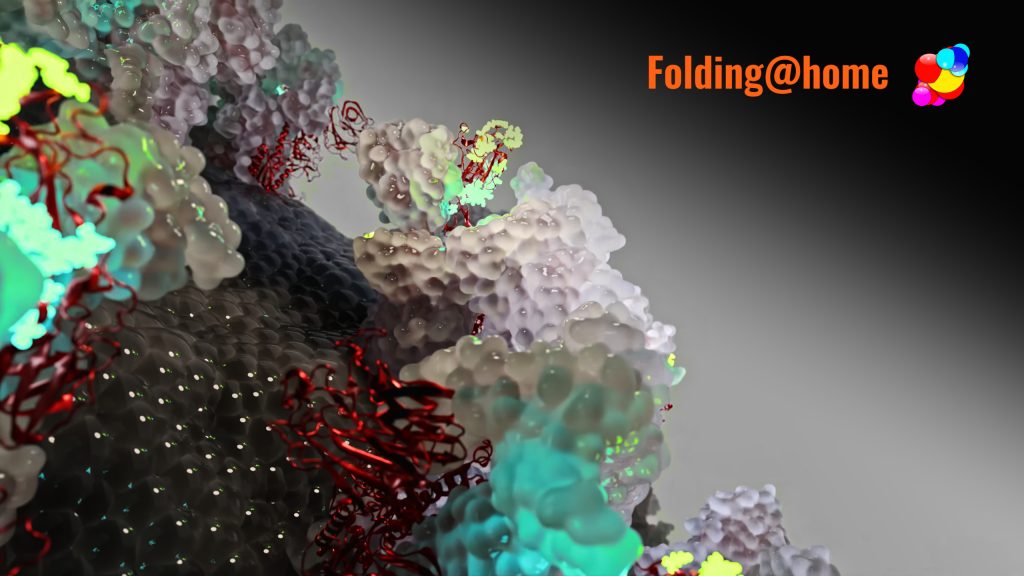
TL;DR: DeepMind’s AlphaFold marks a tremendous advance in our ability to predict the dominant structure of a protein. How proteins get there, and many other problems related to protein folding, remain unsolved. Synergies between AlphaFold and Folding@home could enable more progress.
DeepMind recently claimed they solved the protein folding problem with their AlphaFold algorithm. They made a huge advance, but solving protein folding is a little like saving the world. Many have tried, some have made big steps forward. Yet protein folding won’t stay solved any more than the world will stay saved.
For background, proteins are molecular machines that are responsible for many of the active processes that we associate with life, from muscle contraction to sensing light and digesting food. A protein consists of a chain of chemicals called amino acids, and many “fold” up on themselves into a specific three-dimensional structure that enables them to perform their function.
Protein folding is a huge problem with many parts. One important piece is the structure prediction problem: predicting the dominant structure of a protein from its sequence (a parts list, if you will). Another piece is the folding mechanism problem: how does a protein get to that folded structure? There are also a host of related problems, such as what sort of moving parts do folded proteins have? How do these dynamics enable proteins to transmit information and cargos? How can we design drugs to turn proteins off (or on)? How can we design proteins to perform new functions?
Many people have claimed to solve protein folding. One of my colleagues once gave a really beautiful talk on a discovery they made. After the talk, another colleague quipped, “Congratulations, you solved the protein folding problem! But you still have a long way to go (pointing to yet another colleague), that guy has solved it at least three times.”
DeepMind’s AlphaFold algorithm has leaped ahead of the pack on the protein structure prediction problem. I won’t go into the technical details, but will just say congrats to the team for the big step forward.
With that said, AlphaFold doesn’t explain how proteins fold, which is another important piece of the protein folding problem. It also doesn’t solve the host of other problems that are closely linked, if not part of, the protein folding problem.
Folding@home’s original focus was on understanding how proteins fold into their dominant structures. Since then, the project has broadened its scope to encompass many related problems, since all of these phenomena are driven by the same underlying physical principles.
The general idea behind Folding@home is to harness the power of computers from around the world to simulate protein dynamics. Each simulation is like an explorer. By sending thousands of explorers out in different directions, we’re able to tackle problems that would be intractable on a single computer.
The biomedical applications of Folding@home are quite broad and include Alzheimer’s disease, cancer, and infectious diseases. For example, we are currently focusing much of our attention on understanding the SARS-CoV-2 virus that is responsible for the COVID-19 pandemic. We are also actively engaged in designing new antivirals through the COVID Moonshot.
While AlphaFold hasn’t solved protein folding, it should be an enabling technology. For example, we could use it to predict the dominant structures of proteins whose structures haven’t been determined experimentally. Then we could start simulations on Folding@home from those structures to help understand how the proteins work, following the same procedures we’re currently using to study proteins whose structures have been determined experimentally.
The upshot of all this is that we (the protein science community at large, and Folding@home in particular) still have much work to do! You can lend a hand by downloading/installing Folding@home.